Understanding context can improve experiences between AI and humans
Why context is so significant in AI applications
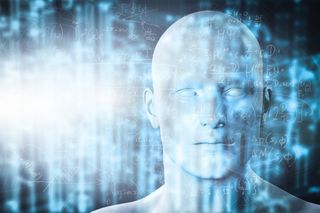
Context is essential in any human interaction. But in the current digital era, grasping and leveraging contextual nuances is paramount for enhancing the quality of our interactions. As we hone these skills, we will increase our ability to revolutionize the future of AI applications. Context encompasses the intricate web of conditions, settings, and factors that shape how we perceive information. We draw insights from situational, personal and social elements and these provide us with the cues we need to understand and interpret messages. Linguistically, context transcends words, delving into the extralinguistic elements that create meaning and comprehension. Without context, meaning can be lost or misunderstood, which underscores its pivotal role.
CPO at Aerospike.
The wide spectrum of context
Context as a concept exists on a wide spectrum that ranges from broad backdrops to granular, in-the-moment specifics. In general, context delivers a foundational understanding; however, in a specific setting, it can be much more nuanced to the particulars of the given situation. For example, when responding to a request while cooking a meal to also prepare a vegetable side dish, the context is not just the food preparation, but consideration of the likes and dislikes of the person making the request. This level of specificity enriches interactions, enabling responses to be modified to individual requirements and circumstances.
When considering AI and context, and in particular, large language models (LLMs), this specificity is achieved using techniques like retrieval-augmented generation (RAG), which integrates detailed contextual cues into queries, producing highly relevant and personalized responses. RAG demonstrates how a nuanced understanding of context can enhance the quality of interaction between humans and AI.
How RAG enhances personalization and context
Context is the most important factor in enabling personalization, particularly in today’s ubiquitous digital environments. The process by which AI assesses a user’s past behavior, their current circumstances and their preferences, allows systems to deliver detailed, highly modified experiences. With AI, the concept of personalization moves past just human interactions to embrace the Internet of Things (IoT) where context is expanded to incorporate variables such as location, environment and historical data.
The role of RAG is to amplify this process by finding and applying specific contextual data from a huge repository of vectors or embeddings. The vectors reflect various facets of a user’s profile or situational data, and they are essential for creating highly relevant responses that are also heavily personalized. As vectors are gathered, they build a library of historical patterns and current status, and this helps to augment the AI’s understanding, allowing it to provide more specific and nuanced responses.
How embeddings work
Embeddings are mathematical representations, also known as vectors, which play a vital role in capturing and making use of context. They work by encoding diverse data aspects, enabling nuanced profiling and semantic searches. The interplay between embeddings and LLMs is cooperative, with embeddings providing a dense contextual setting that helps LLMs with semantic understanding. The outcome is inevitably more precise and more contextually relevant.
As contextual vectors are accumulated – a process called accretion – a more comprehensive understanding starts to develop, and it encompasses various types of interactions, customers, users, or situations. The context that has been assembled to enhance the predictive and responsive capabilities of the AI system relies on the accuracy of the vector search, which is why it is so important to have high-quality, current data to inform these model responses.
Are you a pro? Subscribe to our newsletter
Sign up to the TechRadar Pro newsletter to get all the top news, opinion, features and guidance your business needs to succeed!
Deriving enhanced responses by integrating context in LLMs
Giving contextual cues to LLMs will result in more polished and accurate in-context responses, which are essential for improving user interactions and decision-making. However, the application of context extends beyond the LLM framework, with additional layers of specificity minimizing response variance and ensuring even greater relevance and personalization.
So, let’s look at the capabilities that are needed to implement a system that is so context aware. They start with having a huge, high-throughput vector store. Also needed is efficient ingestion of embeddings that will ensure current context is maintained. The system will also need the ability to generate embeddings from diverse data sources and must have access to models suited for creating and applying embeddings. Finally, the most appropriate foundational model for the task at hand must be selected.
Looking ahead to the next phase of GenAI
In the era of generative AI, context is the cornerstone of valuable and significant interactions and effective decision-making. By gaining comprehension of the nuances of specific, in-the-moment contexts and being able to apply them, AI systems can offer unmatched personalization experiences and relevance, aiding online service providers such as retailers, banks, search engines and streaming companies. There is a synergy between LLMs, RAG, and embeddings that delivers a new paradigm in AI research and application, which promises a landscape in which interactions with AI are as nuanced and understandable as those we currently enjoy amongst ourselves.
We've featured the best AI writer.
This article was produced as part of TechRadarPro's Expert Insights channel where we feature the best and brightest minds in the technology industry today. The views expressed here are those of the author and are not necessarily those of TechRadarPro or Future plc. If you are interested in contributing find out more here: https://anngonsaigon.site/news/submit-your-story-to-techradar-pro
Lenley Hensarling is CPO at Aerospike.